We build
digital products.
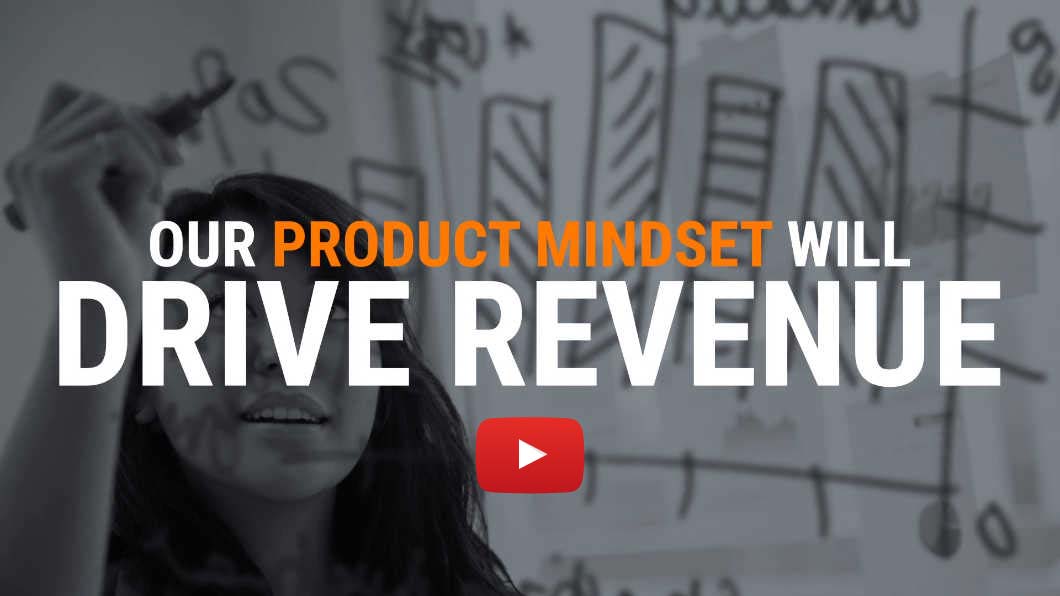
What we do.
Outsourced
Product Development
Digital products are never done. We provide all-inclusive, fully managed product development services for continuous innovation.
Customer Experience Innovation
Develop a deep understanding of your customer’s needs to deliver high value digital experiences.
Platform Modernization
Excel at change by integrating and modernizing your platforms, products, and enterprise operations.
We deliver
extraordinary outcomes
for extraordinary clients.
Brands you know and love partner with 3Pillar Global to understand and expand their business. We’ve helped them grow revenue, increase market share, and boost customer engagement. See the results we’ve achieved working alongside some of our most successful clients.
Read our case studies →
We wrote the book on
The Product Mindset®
The Product Mindset guides our teams to understand your product’s business goals first. This gives them the context they need to build the products that will deliver the business value you seek.
The three principles of The Product Mindset shape how we work.
Solve for Need
Successful products thrive at the intersection of customer and business outcomes. We use fast, focused and flexible methods to listen, watch and learn about your customers to anticipate their needs.
Minimize Time To Value
Value only exists when your customers can touch and experience your product. We build and release in slices that accelerate customer feedback and strengthen stakeholder investment by showing value fast.
Excel at Change
Products are never done. They must adapt to changing customer needs and market conditions. We measure everything we build, communicate transparently, build in iterations, deploy in small pieces and are continuously listening for the need to change.
Get your copy of The Product Mindset
Enter your email below and we will send your digital copy now.